Maximizing Efficiency without sacrificing Effectiveness - Defying the odds in the race to achieve Pe

Selecting an “Efficient” Path to Success
Given the growing sense of urgency and immediate priorities that lie ahead of most public and private sector organizations, and the nation, a critical opportunity exists to properly design and shape the operating model and construct a performance model for organizations that are being consolidated or merged.
Traditionally, organizations that consolidate, take the resources from the newly acquired organization and integrate them based on functions into the existing operating model/construct of the acquirer, providing them with added overall resources to manage and execute tasks followed by what often amounts to “growing pains” as part of the integration. In consolidating or reshaping an organization, often times an organization neglects to select the right track as part of analyzing the vision, mission, and requirements, and more importantly identifying and prioritizing capability gaps at the starting block.
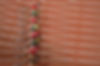
As part of identifying and prioritizing the gaps, an extremely valuable set of analysis that is increasingly being applied both in private and public sector, is Data Envelopment Analysis (DEA), also referred to as bench-marking analysis. As it relates to the M&A process life-cycle or phases, DEA would be applied as a key tool upfront during the first phase of a merger/consolidation, which encompasses activities including but not limited to: Analyzing the Vision, Mission, and Requirements; Identifying and Prioritizing Capability Gaps; and Reviewing the Mergers Integrations & Divestitures strategy. DEA as described in this article, is a tool that aligns well to both process and performance improvement, and the M&A life-cycle with the task of identifying and prioritizing capability gaps, which drives the overall strategy for organizations that are considering merging or consolidating functions.
Data Envelopment Analysis (DEA) is an analytical tool that can assist in the identification of best practices in the use of resources among a group of organizations’. Such identification can highlight possible efficiency improvements that may help organizations or institutions achieve their full potential. Through the use of a representative case study, this article provides a practical guide to developing and refining a DEA model and interpreting of results in the public sector. DEA is a linear programming (LP) technique that identifies the most efficient providers of a service i.e., output by their ability to produce the highest level of a service with a given set of inputs, or to produce given services with the least amount of inputs. DEA was originally developed by Abraham Charnes, William Cooper and Edwardo Rhodes circa 1978 to evaluate nonprofit and public sector organizations. Only recently (i.e. the last two decades) has the private industry such as banking started applying DEA as a method to help identify efficiency gains, successfully identifying millions in excess operating and personnel related costs. The primary goal of DEA is to improve service productivity or organizational performance. With LP as the underlying methodology to DEA, it is commonly used in academia and as a leading analysis technique by operations researchers and statisticians. DEA has not received much attention in many organizations since it requires a more technical/quantitative background to understand, and the source for most DEA related information is academic. DEA can enable leadership and managers to make more informed decisions to improve performance based on bench-marking their own organization or others. The results of DEA deliver performance based data to assist with the reallocation of resources to increase performance of less efficient units, ultimately reducing operational related costs. DEA performs an assessment of input and output variables associated with providing a “service” or meeting a requirement. DEA will help identify the most efficient and equally inefficient business functions to target improvements. Input variables can consist of resources/labor, and output variables; the number of services and requirements satisfied, compared across all functions.
DEAs primary measure is “efficiency,” which can be summarized as a ratio of outputs to inputs. If the maximum amount of output per input is achieved, than the unit being evaluated is considered to be at optimal efficiency. Any additional increase in performance to an optimal efficiency unit would require the implementation of new technology, or newly designed/re-engineered processes. In transaction-based organizations, usually it is not easy to determine “optimum efficiency,” since the relationship between input and output variables is non-discrete, therefore applying DEA will allow for several outputs and inputs to be assessed in order to determine efficiency levels for bench-marking purposes based on several factors including technology, processes, management of processes, et al. DEA is particularly useful in scenarios where leadership is constrained by resources and schedule to implement changes, and multiple outputs and inputs need to be evaluated that have more complex dependencies requiring methods beyond basic regression/correlation analysis, and ratios. To help understand how leadership might apply DEA, we will explore a basic case study of two transaction based organizations that are being consolidated.
Practical Application and Interpretation of DEA

The linear programming technique of DEA is used to find the set of coefficients (u's and v's) that will give the highest possible efficiency ratio of outputs to inputs for the service unit being evaluated. The mathematical model is represented by the following equation:

Subject to (s.t.) the following constraints when the same set of coefficients is being applied across all business units being compared, where no business unit will be more than 100% efficient:

Where in the model:
j = number of business units (BU) being compared in the DBA analysis
BUj = business unit number j
θ = efficiency rating of the business unit being evaluated by DBA
yrj = amount of output r used by service unit j
Xij = amount of input / used by business unit j
ί = number of inputs used by the BUs
r = number of outputs generated by the SUs
ur = coefficient or weight assigned by DBA to output r
vi = coefficient or weight assigned by DBA to input /
Assume that the DEA evaluation would begin by evaluating the efficiency of the transactional organization in table 1-1 below.

For the representative case study consider two separate organizations and various groups or divisions within each being compared represented by Decision Making Units (DMU) referred herein as business units - BU1, BU3, and BU5 for Organization A, and BU2, BU4, and BU6 for Organization B. Each BU processes 150 actions/tasks (e.g., requirements) a month by using two sets of inputs: civil servants measured in full time equivalents (FTE), and methods/tools measured in # of business procedures or processes.
The problem facing leadership related to this case study, is determining the inefficiency levels of each and where to reallocate resources in order to improve performance. Although leadership may be able to compare the numbers in the table, it requires further analysis to understand the efficiency relationship between outputs-to-inputs i.e., FTE and tools/methods needed to satisfy each requirement. Based on the results of the DEA model, it can be observed that BU1, BU2, BU4, and BU5 are relatively inefficient. Each of these business units processed the same number of requirements as BU3 and BU6, however either utilized more FTE or business processes. Therefore these Business Units should be able to reduce the inputs required without impacting the outputs.

Table 1-3 below represents the results of the DEA analysis applied across the sample Business Units for organizations A and B.DEA provides a comparison of each service unit by locating the optimal or units that are not less efficient than other units being evaluated. It also measures the scale of inefficiency compared to the optimal units. The optimal units are relatively efficient and are identified by a DEA efficiency rating of Θ = 1. The inefficient units are identified by an efficiency rating of less than 1 (Θ < l). DEA will provide an efficiency rating between 0 and 1, which is referred to as an efficiency percentage using a range of 0 and 100%. The upper limit is set as 1 or 100% to reflect the view that a unit cannot be more than 100% efficient.
DEA identified inefficient business units that were identifiable through observation of the data. BUl, BU2, BU4, and BU5 have efficiency ratings below 100% denoted by the Objective Value column in the table 1-2, which identifies them as inefficient. In addition, DEA provides insight to leadership to a subgroup known as an efficiency reference set (ERS) which are the relative weights or lambda values of BU3 and BU6 denoted in the two right hand columns table 1-3. This efficiency reference set (ERS) includes the set of business units against which each inefficient business unit was found to be most directly inefficient. For example, BU4 was found to have operating inefficiencies in direct comparison to BU3 and BU6. The lambda/ERS value represents the relative weight assigned to each inefficient BU to calculate the efficiency rating (Θ). The graphical representation of the business units in Figure 1-2 illustrates this by using BU4 as an example. If a business units efficiency rating is 100%, then this unit is its own ERS and therefore is not typically reported, which is why BU3 and BU6 do not have an ERS reported.

DEA has determined that, among the six business units, BU3 and BU6 are relatively efficient. In this case study example, the solid line in Figure 1-1, which locates the units that used the least amount of inputs to produce their output level, represents this. These two business units comprise the best practice set or efficiency frontier. Additionally, no indication is provided regarding which BU is more or less efficient. It is important to note that B3 and B6 could also be somewhat inefficient, however as applied in DEA the optimally efficient business units are considered those that are not clearly inefficient in comparison with other business units.

DEA indicates that BU4 is inefficient compared to point e on the line between BU3 and BU6 in Figure 1-1. One way for BU4 to become efficient is for it to reduce its inputs to 75% of its current level. This would move BU4 to the relatively efficient production frontier at point e in Figure 1-1, which reflects the use of 37.5 FTE (0.75 x 50) and use of 11 methods/tools i.e. business processes (0.75x 15). DEA provides information to complete the calculation as performed in Tables 1-4 and 1-5 below. (In the table, the inputs and outputs of business units - BU3 and BU6 are multiplied by the weights (lambda values) derived by DEA described earlier, 0.917 and 0.083 respectively. These are then added together to create a future state or “To-Be” business unit that provides equal or greater output as the inefficient business unit, B4, while using less inputs.

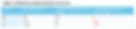
The results of the DEA in the above tables indicates that a mix of best practices/techniques from the efficient sets (BU3, and BU5) would lead to the formation/restructuring of a Business Unit that produces the same number of requirements with less resources and tools/methods i.e. business processes. If the same technique is applied across all other inefficient business units identified, the projected “To-Be” state can be achieved as summarized by the DEA output in Table 1-6 below.

In summary, applying DEA is most useful when there are multiple outputs and inputs, and when intuitive analysis that could be applied to verify the DEA results (unlike this case study) would not be possible. Further, all data driven results demonstrated as part of this case study are readily available to leadership as part of applying DEA and include the efficiency rating, the efficiency reference set, analysis performed to determine “To-Be” impacts, and the ability to determine alternative paths that would make an inefficient unit efficient. Lastly, leadership has the ability to leverage multiple open source and existing software tools including Microsoft Office based tool suites coupled with experienced professionals that have backgrounds in fields such as performance improvement, statistics, industrial/systems engineering, and mathematics that will enable them to take advantage of performing this type of analysis. The end result of applying this risk adverse method is better informed leadership decisions on where additional considerations or questions need to be asked that will result in a more efficient use of resources while providing the same desired outputs i.e., doing more with less.
Finishing the Race
As budgets tighten and pressures surmount to target further efficiencies across the public and private sector; austerity measures, and institutional reform like initiatives including shared services, consolidation/mergers of agencies and organizations, and program shut downs will all largely remain a common response tactic of leadership as they seek opportunities to reduce spend. This is not the first time and will not be the last that organizations will be faced with having to make major financial decisions with an outcome that historically has resulted in the slowing the growth of programs that end up being funded later at additional costs, far exceeding what was originally planned.

In conclusion, as part of achieving the goal of optimizing performance and overall efficiency, the objectives of broader public and private sector initiatives, must carefully consider the right level analysis has been performed upfront to guide decisions based on supporting facts and evidence vice intuition and relationships. Applying performance improvement methods, tools and techniques such as DEA by leveraging available technologies coupled with experienced professionals provides organizations with proven analytical methods to target efficiencies in a smarter way. Data envelopment analysis is a technique that can be used to assist in identification of best practice performance in the use of resources, highlighting where the greatest gains may be made from improvements in efficiency, and helping organizations achieve their full potential. This approach provides a starting point in an organizations race to perform, and at the finish will lead to outcomes including: cutting waste; eliminating duplication, overlap, and unnecessary overhead costs; and more efficiently and effectively serving the organization and its customers by maximizing the use of personnel and financial resources.
As always, I'm interested to hear about your experiences with cost savings initiatives across organizations and the challenges with realignments, mergers, integrations, and consolidation efforts. What methods have you applied to achieve optimal outcomes?
Please feel free to like and share the article, or submit any questions you might have on this topic.
References
Sherman, H.D. and J. Zhu, “Service Productivity Management Improving Service Performance using Data Envelopment Analysis (DEA)”, Springer 2006
Commonwealth of Australia, “DEA - A Technique for Measuring the Efficiency of Government Service Delivery,” Melbourne, 1997
Wilson, P.W. 1995, “Detecting influential observations in data envelopment analysis”, The Journal of Productivity Analysis, vol. 6, pp. 27-45